- Out-of-Stock
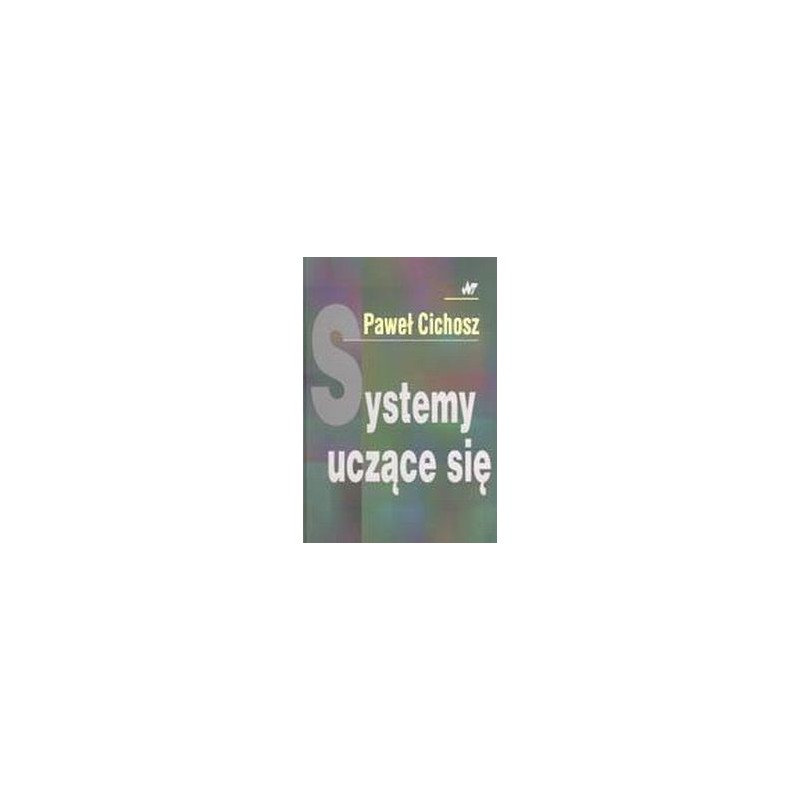
free shipping in Poland for all orders over 500 PLN
If your payment will be credited to our account by 11:00
Each consumer can return the purchased goods within 14 days
Table of Contents
1 Learning in an algorithmic approach
2 Induction learning
3 Induction of decision trees
4 Rule induction
5 Probabilistic methods
6 Conceptual grouping
7 Transforming attributes
8 Learning the function approximation
9 Inductive logic programming
10 Making discoveries
11 Learning by explaining
12 Learning machines
13 Learning with reinforcement
14 Termination
No product available!
No product available!
No product available!
No product available!
XTREME DT830B multimeter enabling constant voltage measurement in the range of 200 mV - 500 V, alternating voltage 200 - 500 V, direct current 200 μA - 5 A and resistance 200 Ω - 2 MΩ. DT830B XTREME
No product available!
Single-board computer with Samsung S3C2440 processor (ARM9) with 7 inch LCD and touchpanel
No product available!
No product available!
No product available!
No product available!
No product available!
No product available!
16 Mpx camera module with IMX298 sensor compatible with all versions of Raspberry Pi. The maximum video resolution is 4672×3496 pixels. ArduCAM B0174
No product available!
No product available!
Banana Pi M2+ is another ARM SoC powered development board that offers great computing performance in an ultra portable form factor. It is a 65mm square with Allwinner H3 Quad-core A7 SoC and 1GB DDR3 RAM
No product available!
No product available!
No product available!